RETRACTED: PSCNN: PatchShuffle Convolutional Neural Network for COVID-19 Explainable Diagnosis
Objective: COVID-19 is a sort of infectious disease caused by a new strain of coronavirus. This study aims to develop a more accurate COVID-19 diagnosis system.
Methods: First, the n-conv module (nCM) is introduced. Then we built a 12-layer convolutional neural network (12l-CNN) as the backbone network. Afterwards, PatchShuffle was introduced to integrate with 12l-CNN as a regularization term of the loss function. Our model was named PSCNN. Moreover, multiple-way data augmentation and Grad-CAM are employed to avoid overfitting and locating lung lesions.
Results: The mean and standard variation values of the seven measures of our model were 95.28 ± 1.03 (sensitivity), 95.78 ± 0.87 (specificity), 95.76 ± 0.86 (precision), 95.53 ± 0.83 (accuracy), 95.52 ± 0.83 (F1 score), 91.7 ± 1.65 (MCC), and 95.52 ± 0.83 (FMI).
Conclusion: Our PSCNN is better than 10 state-of-the-art models. Further, we validate the optimal hyperparameters in our model and demonstrate the effectiveness of PatchShuffle.
CITE THIS COLLECTION
REFERENCES
- https://doi.org//10.22034/iji.2021.87723.1824
- https://doi.org//10.3201/eid2608.201843
- https://doi.org//10.1148/radiol.2020200642
- https://doi.org//10.7759/cureus.9448
- https://doi.org//10.1016/j.compbiomed.2020.103805
- https://doi.org//10.1148/radiol.2020200905
- https://doi.org//10.1109/TMI.2020.2995965
- https://doi.org//10.1007/978-981-15-9682-7_8
- https://doi.org//10.1109/ACCESS.2020.3028012
- https://doi.org//10.1007/978-981-15-9682-7_6
- https://doi.org//10.32604/cmc.2021.018040
- https://doi.org//10.1016/j.ijcce.2021.05.001
- https://doi.org//10.1007/978-3-030-84532-2_45
- https://doi.org//10.32604/cmes.2021.015807
SHARE
Usage metrics
Read the peer-reviewed publication
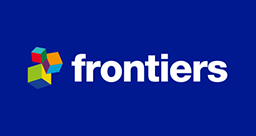
AUTHORS (3)
- SWShui-Hua WangZZZiquan ZhuYZYu-Dong Zhang
CATEGORIES
- Aboriginal and Torres Strait Islander Health
- Aged Health Care
- Care for Disabled
- Community Child Health
- Environmental and Occupational Health and Safety
- Epidemiology
- Family Care
- Health and Community Services
- Health Care Administration
- Health Counselling
- Health Information Systems (incl. Surveillance)
- Health Promotion
- Preventive Medicine
- Primary Health Care
- Public Health and Health Services not elsewhere classified
- Medicine, Nursing and Health Curriculum and Pedagogy
- Nanotoxicology, Health and Safety
- Mental Health Nursing
- Midwifery
- Nursing not elsewhere classified