Detection of arterial remodeling using epicardial adipose tissue assessment from CT calcium scoring scan
Non-contrast CT calcium scoring (CTCS) exams have been widely used to assess coronary artery disease. However, their clinical applications in predicting coronary arterial remodeling remain unknown. This study aimed to develop a novel machine learning model to predict positive remodeling (PR) from CTCS scans and evaluate its clinical value in predicting major adverse cardiovascular events (MACE).
MethodsWe analyzed data from 1,324 patients who underwent both CTCS and CT angiography. PR was defined as an outer vessel diameter at least 10% greater than the average diameter of the segments immediately proximal and distal to the plaque. We utilized a total of 246 features, including 23 clinical features, 12 Agatston score-derived features, and 211 epicardial fat-omics features to predict PR. Feature selection was performed using Elastic Net logistic regression, and the selected features were used to train a CatBoost machine learning model. Classification performance was evaluated using 1,000 repetitions of five-fold cross-validation and survival analyses, comparing actual and predicted PR in the context of predicting MACE.
ResultsPR was identified in 429 patients (32.4%). Using Elastic Net, we identified the top 13 features, including four clinical features, three Agatston score-derived features, and six fat-omics features. Our method demonstrated excellent classification performance for predicting PR, achieving a sensitivity of 80.3 ± 1.7%, a specificity of 89.7 ± 1.7%, and accuracy of 81.9 ± 2.5%. The Agatston-score-derived and fat-omics features provided additional benefits, improving classification performance. Furthermore, our model effectively predicted MACE, with a hazard ratio (HR) of 4.5 [95% confidence interval (CI): 3.2–6.4; C-index: 0.578; p < 0.00001] in the training set and an HR of 3.2 (95% CI: 2.5–4.0; C-index: 0.647; p < 0.00001) in the external validation set.
ConclusionWe developed an innovative machine learning model to predict coronary arterial remodeling from epicardial fat and calcification features from low-cost/no-cost screening CTCS scans. Our results suggest that vast number of CTCS scans can support more informed clinical decision-making and potentially reduce the need for invasive and costly testing for low-risk patients.
CITE THIS COLLECTION
REFERENCES
- https://doi.org//10.1016/S0140-6736%2818%2932203-7
- https://doi.org//10.1093/eurheartj/ehu299
- https://doi.org//10.1161/CIR.0000000000001029
- https://doi.org//10.1148/radiol.213271
- https://doi.org//10.1016/j.jcct.2024.04.009
- https://doi.org//10.1016/j.jcmg.2023.05.020
- https://doi.org//10.1016/j.jacc.2018.10.066
- https://doi.org//10.1016/j.atherosclerosis.2020.03.019
- https://doi.org//10.1016/j.jacl.2020.12.005
- https://doi.org//10.1016/j.jcmg.2022.06.018
- https://doi.org//10.1038/s41598-024-60584-8
- https://doi.org//10.1056/NEJMoa1805971
- https://doi.org//10.1016/S0140-6736%2815%2960291-4
- https://doi.org//10.1016/j.jacc.2016.02.026
SHARE
Usage metrics
Read the peer-reviewed publication
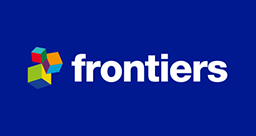
AUTHORS (10)
- JLJuhwan LeeTHTao HuMWMichelle C. WilliamsAHAmmar HooriHWHao WuJKJustin N. KimDNDavid E. NewbyRGRobert GilkesonSRSanjay RajagopalanDWDavid L. Wilson